The GDPR framework issued by the European Union has created its fair share of impact on all firms dealing with some form of data in Europe. Many people might think that the GDPR is just an IT issue, but that is the furthest statement from the truth. It has broad-sweeping implications for the whole company, including the way companies handle marketing and sales activities. However, the implications of this framework are not geographically bound by Europe.
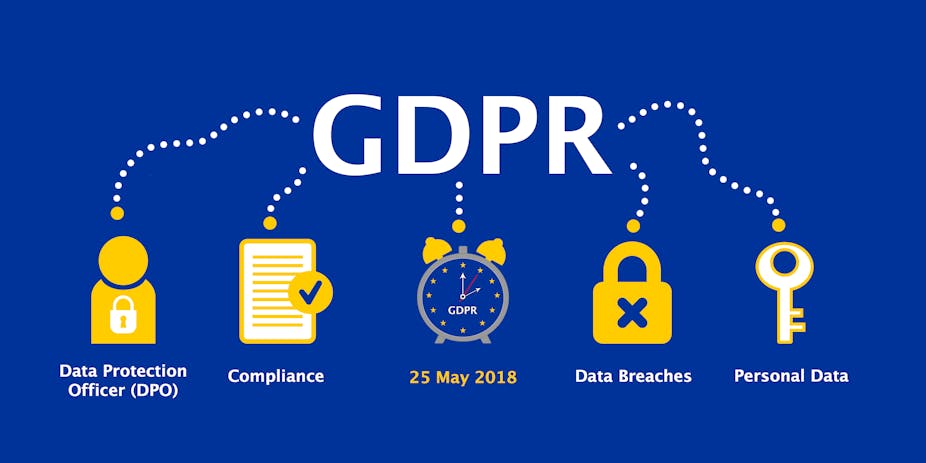
U.S. news sites have had a different experience with GDPR than European sites. Two months after the law’s enforcement, more than 1,000 news publishers chose to block European visitors from their sites. According to research from ad tech firm Catchpoint.Ad rates have increased 10 per cent in the U.S. since May 25 and dropped in Europe, according to research from analytics firm Ezoic.
Rational economic actors will respond in predictable ways to manage such risk. Data-Backed Securities (DBS) might allow organisations to convert unpredictable future revenue streams into one single payment. Collateralised Data Obligations (CDO) might allow data collectors to package personal data into tranches of varying risk of consent withdrawal. A secondary data derivative market is thus created — one that we have very little idea of how it will operate, and what any secondary effects may be.
With almost a year gone by, most people have forgotten the pivotal impact created by GDPR on regulating data collection. The article is written from a perspective that would mainly appeal to professionals who casually know about this regulation.
- Rohan Bhasin
Informative
ReplyDelete